What Is The Most Common Problem In AI?
Artificial Intelligence (AI) is becoming an increasingly important part of our lives, with new applications being developed every day. However, AI is still in its early stages and there are many challenges that still need to be overcome. One of the most common problems in AI is the lack of understanding of how to create machines that can learn and make decisions on their own. AI is heavily reliant on large datasets and algorithms to make decisions, but these algorithms can sometimes be too general or even inaccurate. This can lead to machines making decisions that are not in the best interest of the user or the environment. Additionally, AI systems can be prone to bias due to the data they are trained on, and this can lead to inaccurate predictions or decisions. Finally, another common problem is the lack of trust that users have in AI systems, as they can be difficult to understand for non-specialists.
Overview of AI
Artificial Intelligence (AI) is a form of computer science that enables machines to learn from experience and act in a manner similar to humans. As AI-powered machines become increasingly capable, they are being used to automate tasks, solve complex problems, and provide insights that traditional computing solutions can’t. AI is being applied to a wide range of industries, from healthcare and finance to manufacturing and retail.
However, AI is not without its challenges. Complex algorithms and data sets must be developed and managed in order to make AI work. In addition, there are ethical and regulatory decisions that must be made in order to ensure that AI is not misused or abused. The most common problem in AI is that it can be difficult to predict how a machine will react to a certain situation or environment. This unpredictability can lead to unexpected results, and can potentially create issues for companies that use AI in their operations.
There are also concerns about AI’s potential to cause job displacement and the need to develop safeguards and regulations to ensure its safe and ethical use. Furthermore, AI systems must be designed to be resilient to cyberattacks, requiring secure coding practices and robust data security measures.
Overall, AI promises to revolutionize many industries. However, to make sure that it is used effectively and safely, companies must be aware of the potential issues and challenges associated with AI. Proper planning and risk management will be essential to ensure that AI is used responsibly and ethically.
Types of Common AI Problems
Artificial Intelligence (AI) is a rapidly developing field that has seen a surge in research and development in recent years. As AI applications become more complex and sophisticated, so too do the challenges associated with them. From data mining and automation to machine learning and natural language processing, AI can be applied to a variety of areas. While the potential for AI is vast, it is also important to consider the common problems associated with it.
Some of the most common issues in AI include the lack of data availability, the difficulty of creating accurate models, the difficulty of interpreting results, and the potential for bias in AI systems. Data availability is a major issue as AI relies on data to generate models and make predictions. Without adequate data, AI systems will be unable to make accurate predictions or learn from past experiences. Similarly, creating accurate models is difficult due to the complexity of the data. AI systems must be able to interpret data accurately in order to make accurate predictions, but this can be a challenge due to the complex nature of the data.
The potential for bias is another major issue in AI. AI systems are only as good as the data they are trained on, and if the data is biased or contains errors, the AI system will be too. This can lead to inaccurate predictions and results. Finally, interpreting the results of AI systems is a challenge due to the complexity of the data and the models used to generate the results. AI systems may provide accurate results, but without an understanding of how the system reached its conclusions, the results may be difficult to interpret.
In conclusion, AI is a rapidly developing field with many potential applications. However, there are common issues associated with AI such as data availability, model accuracy, potential for bias, and difficulty of interpreting results that should be addressed in order to ensure the success of AI systems.
Impact of AI Problems
From self-driving cars to facial recognition systems, artificial intelligence (AI) is quickly becoming an integral part of our everyday lives. However, as with any technology, AI has its own set of challenges. AI problems can range from technical issues such as data accuracy and computing power to ethical issues such as privacy and safety. This article will explore the most common problems in AI, including the impact they can have on both businesses and society.
A major issue with AI is that it can often produce inaccurate results due to lack of data or inadequate data quality. This can lead to incorrect predictions and decisions that have far-reaching consequences. For example, facial recognition algorithms have been found to misidentify people of color more often than white people, leading to potential discrimination. Additionally, AI algorithms can be biased due to biased data sets, resulting in decisions that are unfair or even discriminatory.
Another problem is the lack of transparency in AI systems. AI algorithms often operate on complex datasets, making it difficult for even experts to understand how they are making decisions. This lack of transparency can lead to situations where users are unaware of their rights and responsibilities when using AI systems, and can also lead to companies overstepping their boundaries and misusing user data.
Finally, AI systems can also open up new security vulnerabilities. AI systems can be vulnerable to attack, and malicious actors can use AI to create targeted attacks on businesses or individuals. In addition, AI systems can also be used to manipulate data, leading to the potential for fraud and identity theft.
Overall, AI presents many challenges that must be addressed in order to ensure that it is used responsibly. From data accuracy to ethical issues, there are a number of issues that must be addressed in order to ensure that AI is used safely and ethically. By understanding the most common problems in AI, businesses and individuals can work to ensure that AI is used responsibly and ethically, and that its potential is fully realized.
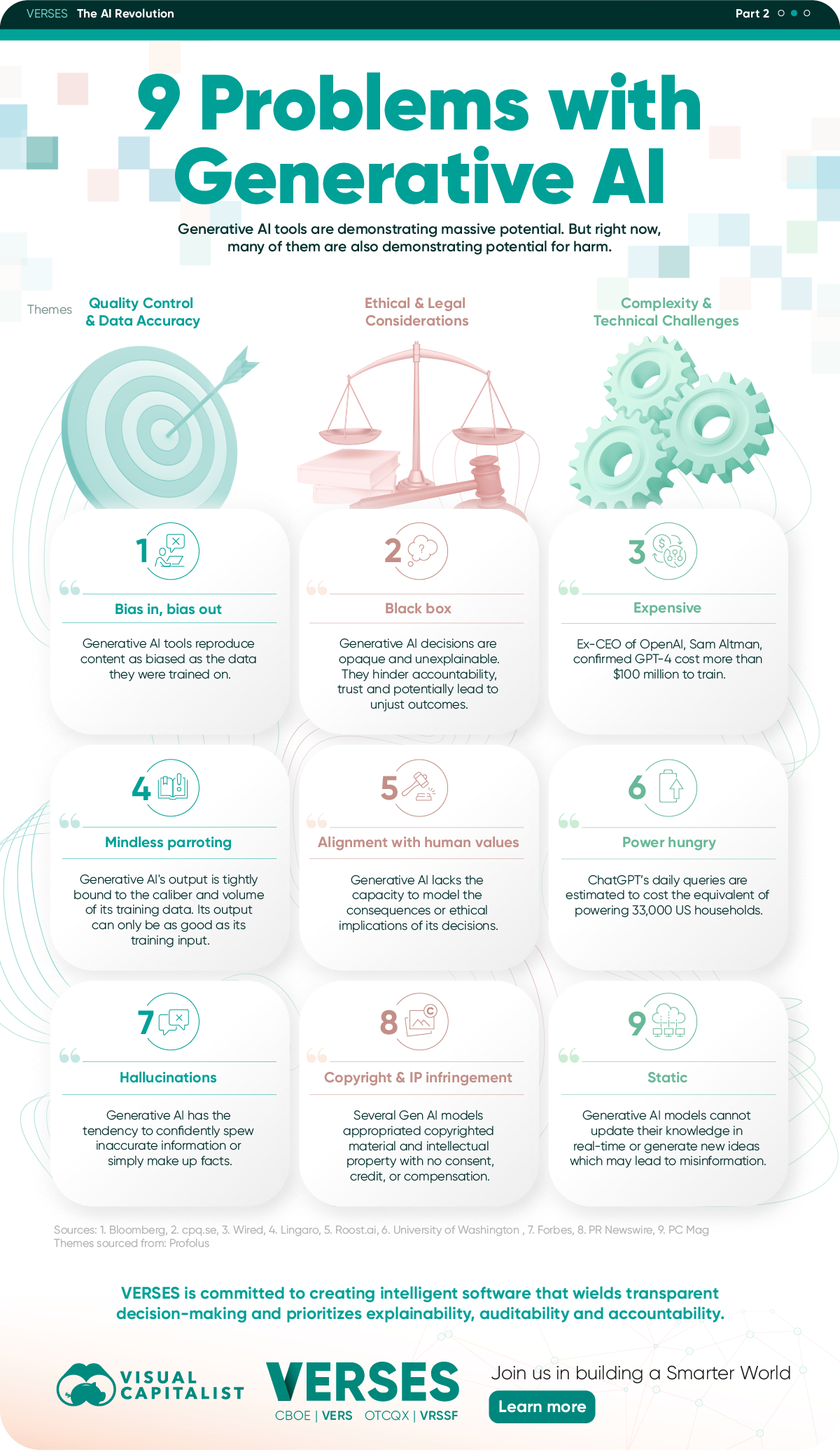
Solutions to AI Problems
AI is a rapidly developing field with numerous potential applications in a wide range of industries. However, there are some common issues that may impede the development and implementation of AI solutions. In this article, we will discuss the most common problems in AI, and how they can be addressed.
The most common problem in AI is the lack of data. AI requires large datasets in order to learn and develop more accurate models. Obtaining the necessary data can be a difficult and expensive task. To address this issue, researchers are exploring ways to use synthetic data or transfer learning to train AI systems.
Another issue is the lack of AI talent. AI requires highly skilled professionals who understand the principles of machine learning and deep learning. However, the demand for these professionals is greater than the supply, making it difficult for organizations to find the necessary talent. To address this problem, organizations should invest in training and development programs to help existing employees gain the skills they need to work with AI.
Finally, AI systems require significant computing power to run complex algorithms and process large datasets. Organizations must invest in powerful hardware and cloud solutions in order to run their AI systems.
In summary, the most common problems in AI include a lack of data, a lack of AI talent, and a lack of computing power. To address these issues, organizations should consider using synthetic data, transfer learning, training and development programs, and powerful hardware and cloud solutions. By taking these steps, organizations can ensure that their AI projects are successful.
Challenges in Solving AI Problems
Artificial Intelligence (AI) is a rapidly evolving field that is quickly becoming a major part of our everyday lives. With the ever-increasing reliance on AI, the potential for failure in operations is an ever-present risk. To ensure that AI systems are reliable and effective, it is important to identify and address the most common problems in AI.
One of the most common problems in AI is the difficulty of solving AI problems. AI problems are extremely complex and require the use of sophisticated algorithms and techniques. This complexity can make it difficult to identify and solve the underlying problems, leading to inefficient or incorrect solutions. Furthermore, AI systems often require massive datasets for training and may not be able to learn from small datasets. This can lead to large resource requirements and slow training times, making it difficult to solve AI problems in a timely manner.
Another common problem in AI is the lack of interpretability of AI models. AI models are often very complex and difficult to interpret, making it difficult to understand how the model is making decisions and identify where errors may be occurring. This lack of interpretability makes it difficult for developers to debug and optimize the system.
Finally, AI systems often struggle with generalizing from data. AI algorithms often require large amounts of data to generalize effectively. Without enough data, the model may not be able to effectively generalize, leading to poor performance.
Overall, AI systems are incredibly powerful and have the potential to revolutionize many industries. However, there are several common problems in AI that must be addressed to ensure reliability and effectiveness. These include difficulty in solving AI problems, lack of interpretability, and difficulty in generalizing from data. With the proper methods and techniques, these problems can be addressed and AI systems can become even more powerful.
The Future of AI Problem-Solving
As technology continues to develop, Artificial Intelligence (AI) is becoming an increasingly important part of our world. AI is being used to solve problems in a range of industries such as agriculture, healthcare, finance, and transportation. However, despite the huge potential of AI, there are still many challenges that it must overcome. One of the most common problems in AI is the difficulty of problem-solving.
AI is capable of performing complex tasks, but it still lacks the ability to effectively solve problems in the same way humans do. Traditional problem-solving techniques rely heavily on the use of specific algorithms, which can be difficult to apply to more complex problems. AI research is now focused on developing more advanced problem-solving techniques that are better able to handle the complexities of the real world.
One approach to problem-solving in AI is the use of deep learning. Deep learning is a form of machine learning that uses neural networks to process data and identify patterns. This allows AI to create more complex models that are better able to identify and solve problems.
Another approach to problem-solving in AI is the use of evolutionary algorithms. These algorithms use natural selection and genetic algorithms to create solutions to problems. This approach is especially useful for solving problems that are too complex for traditional algorithms.
The future of AI problem-solving is incredibly exciting. By combining deep learning and evolutionary algorithms, AI can be used to solve more complex problems than ever before. This will open up new possibilities for AI, allowing it to tackle problems in a range of industries and to create innovative solutions.
FAQs About the What Is The Most Common Problem In AI?
1. What types of problems are most commonly encountered in AI?
The most common problems encountered in AI are related to data, algorithms, and optimization. Data problems include data cleaning, feature engineering, and data integration. Algorithm problems include selecting the right model for the task, finding ways to improve model accuracy, and adapting models to new data. Optimization problems include hyperparameter optimization and finding ways to speed up training times.
2. How difficult is it to solve common problems in AI?
The difficulty of solving common problems in AI varies significantly depending on the task and the data available. In some cases, basic algorithms may be enough to solve the problem, while in other cases, more complex models will be needed. Additionally, the difficulty of solving the problem can increase significantly depending on the size and complexity of the data.
3. What tools are available to help solve common problems in AI?
There are a variety of tools available to help solve common problems in AI. These can range from basic libraries and frameworks such as TensorFlow and Scikit-learn to more specialized tools such as AutoML and reinforcement learning platforms. Additionally, there are a variety of online resources and tutorials available to help get started with AI.
Conclusion
The most common problem in AI is that of overfitting. Overfitting occurs when an AI model is too complex and is unable to generalize well to new data. This can result in poor performance when the AI is presented with data it hasn’t seen before. To prevent this, it is important to use techniques such as regularization or cross-validation to ensure the AI model is able to generalize well. Additionally, it is important to use datasets that contain a wide range of data in order to better test the model’s ability to generalize.